Reveal Stratigraphy through Machine Learning
Reveal Stratigraphy
Using the Paradise® AI workbench, geoscientists can generate and analyze seismic data at the sample level, well beyond a wavelet. This powerful capability, along with the application of machine learning to Multi-Attribute Classification, produces profound, sometimes surprising results, particularly the ability to detect features below conventional seismic tuning thickness. Attribute Selection is done through the use of Principal Component Analysis (PCA), and Multi-Attribute Classification is based on the highly robust Self-Organizing Map (SOM) technique, which is applicable with or without well control. These machine learning tools are applicable in both exploration and production.
Using the Paradise AI workbench, geoscientists can generate and analyze seismic data at the sample level, well beyond a wavelet. This powerful capability, along with the application of machine learning to Multi-Attribute Classification, produces profound, sometimes surprising results, particularly the ability to detect features below conventional seismic tuning thickness. Attribute Selection is done through the use of Principal Component Analysis (PCA), and Multi-Attribute Classification is based on the highly robust Self-Organizing Map (SOM) technique, which is applicable with or without well control. This means these tools in the workbench are appropriate for both exploration and production.
Stratigraphic Analysis ThoughtFlow® in Paradise
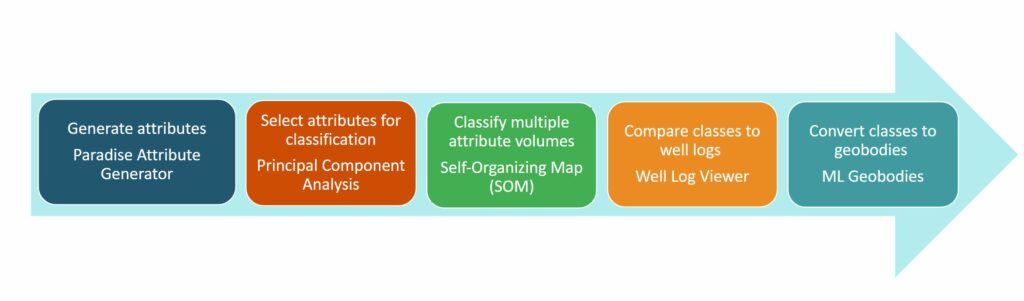
The Stratigraphic Analysis ThoughtFlow in Paradise allows interpreters to:
Highlight reservoirs and stratigraphic features at the sample level
Detect thin beds and direct hydrocarbon indicators (DHIs)
Compare machine learning results to well logs to refine results
Pictured Above: Resolution comparison between conventional seismic display and a Paradise multi-attribute SOM classification. (left) Seismic amplitude profile through the 6 well and (right) the SOM results of the same profile identifying the Eagle Ford group that comprises 26 sample-based neuron clusters, which are calibrated to facies and systems tracts. The 2D color map displays the associated neuron cluster colors. Seismic data owned by and provided courtesy of Seitel Inc.
“This study demonstrates a clear improvement in reservoir characterization compared to the traditional QI approach.”
– Sharareh Manouchehri, et al., Wisting Case Study, First Break, 2020
Supporting resources on stratigraphic analysis using Paradise
Learn how geoscientists are using the Machine Learning Stratigraphic Analysis in Paradise to generate and analyze seismic data at the sample level, well beyond a wavelet.
Applying Unsupervised Multi-Attribute Machine Learning for 3D Stratigraphic Facies Classification in a Carbonate Field, Offshore Brazil
Applying ML Technologies in the Niobrara Formation, DJ Basin, to Quickly Produce an Integrated Seismic Classification Volume
A Multi-Disciplinary Approach to Establish a Workflow for the Application of Machine Learning for Detailed Reservoir Description
Step 1: Discovery – Schedule Now
Schedule your introductory call to discuss what AI can do for you.
Start your AI implementation journey today with a short, 30-minute introductory call. We will listen carefully to your objectives and design a path for your team that realizes tangible benefits.