AI-Assisted Fault Detection in Paradise® – Now with Custom Training Lines
AI-Assisted Fault Detection in Paradise® – Now with Custom Training Lines
Fault interpretation is one of the most time-intensive and subjective steps in seismic analysis. Paradise® accelerates this process with AI-Assisted Fault Detection — a robust, machine learning-driven workflow that enhances interpreter efficiency while preserving full control over the interpretation. Now in version 3.6.2, you can go beyond automation: choose your own seismic lines as training data, blending expert judgment with the power of AI.
Interpreters spend a lot of time identifying faults in seismic data by picking 2D lines. This critical process can now be automated with AI Fault Detection in Paradise, which uses deep learning and machine learning processes to generate fault volumes for fault interpretation. Check out the short video to the right to see results from AI Fault Detection, including for complex fault regimes or noisy seismic data. More examples of Fault Detection results are shown below. Select the technical papers below to learn more about fault interpretation and automatic Fault Detection.
AI That Works With You, Not Instead of You
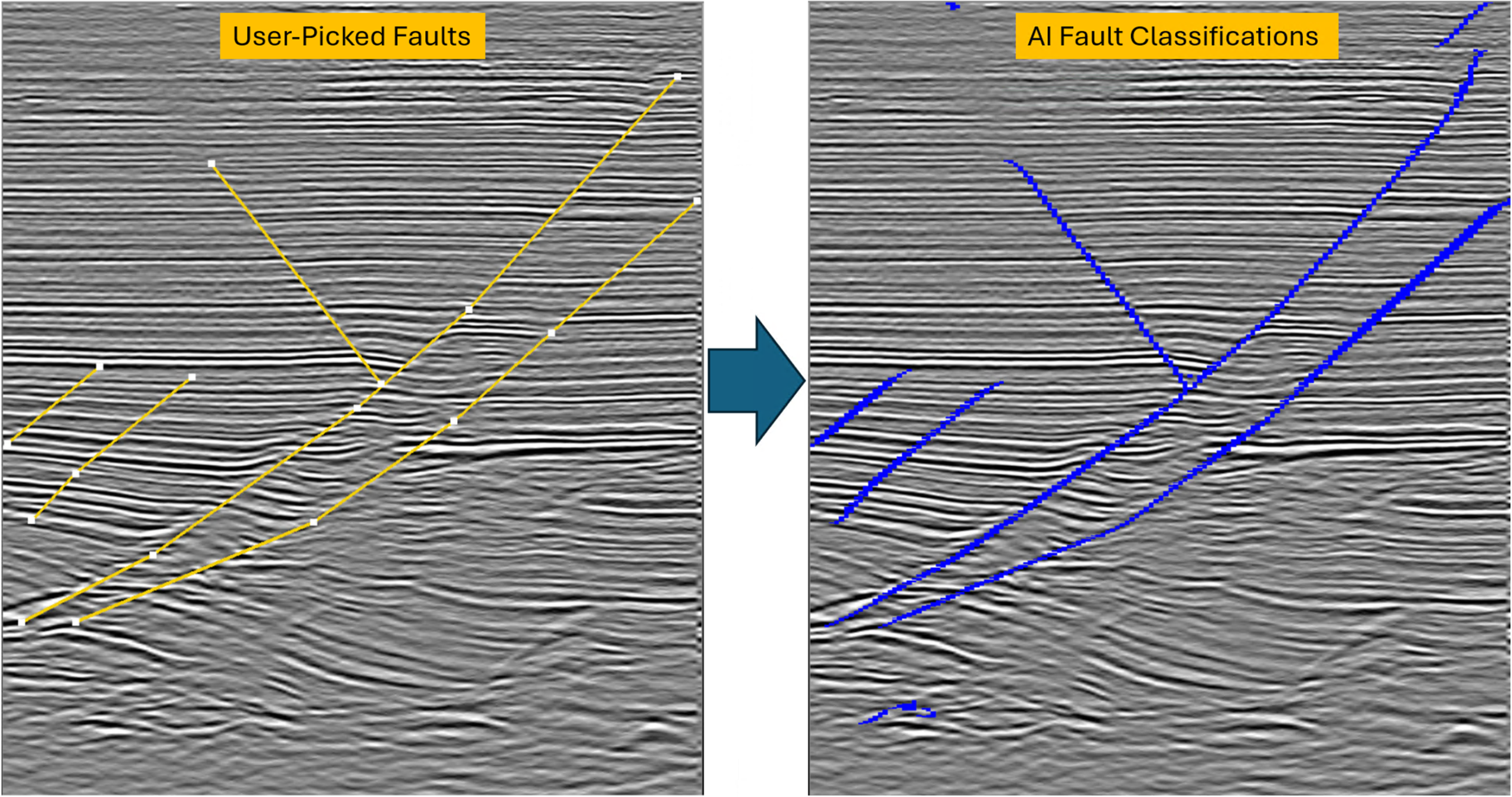
Whether you’re working in noisy data, complex fault regimes, or looking to reduce interpretation time across a large 3D volume, the Paradise hybrid approach delivers. Rather than replace the interpreter, Paradise amplifies your expertise by automating repeatable steps and exposing structures that may be missed in traditional workflows.
Key features of AI Fault Detection in Paradise 3.6.2:
- User-defined training input: Select your own lines to guide AI detection
- Deep learning with 3D CNN architecture for fault probability extraction
- Integrates expert picks with synthetic training data to balance control and consistency
- Export-ready fault volumes for geomodeling and structural workflows (e.g., fault planes in Petrel)
Faster Interpretation. Greater Control.
At the core of Paradise’s fault detection is a powerful semi-supervised deep learning engine, trained on 3D synthetic seismic fault models. In earlier versions, interpreters benefited from pre-trained models that quickly produced fault probability volumes without requiring manual picks. With 3.6.2, Paradise evolves to meet the needs of users who want more customization: you can now guide the learning process by selecting representative inline and crossline sections to define fault characteristics. The AI incorporates this input to deliver more geologically relevant, high-resolution results—tailored to your basin and seismic style.
This capability offers the best of both worlds:
- Speed and scalability through AI-powered workflows
- Geological accuracy through expert-informed training data
- Transparency and flexibility in tuning the model to your interpretation standards
See it in action
Schedule your introductory call to discuss what AI can do for you.
Explore the results of AI Fault Detection in our short demo video, or contact our team for a hands-on walkthrough using your data.